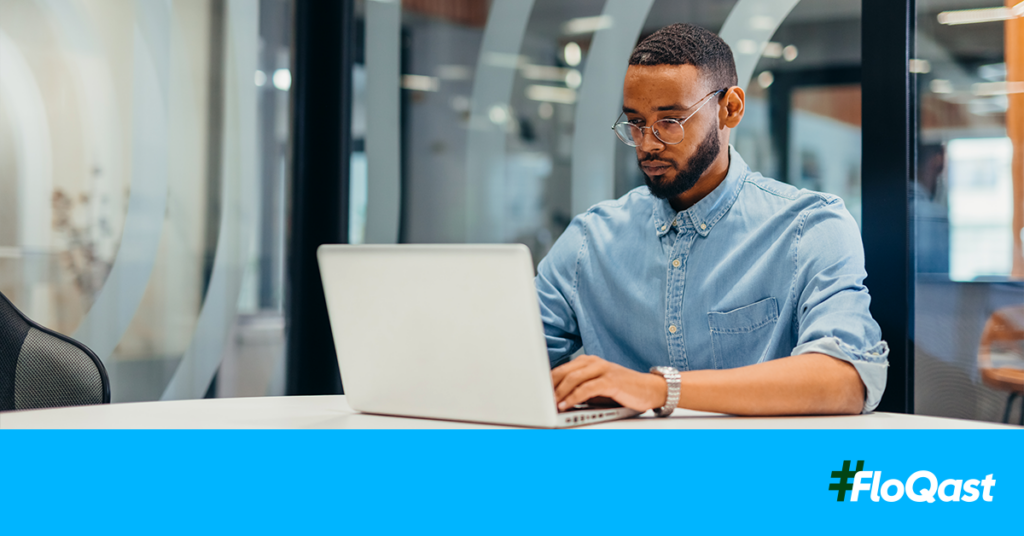
Blog -
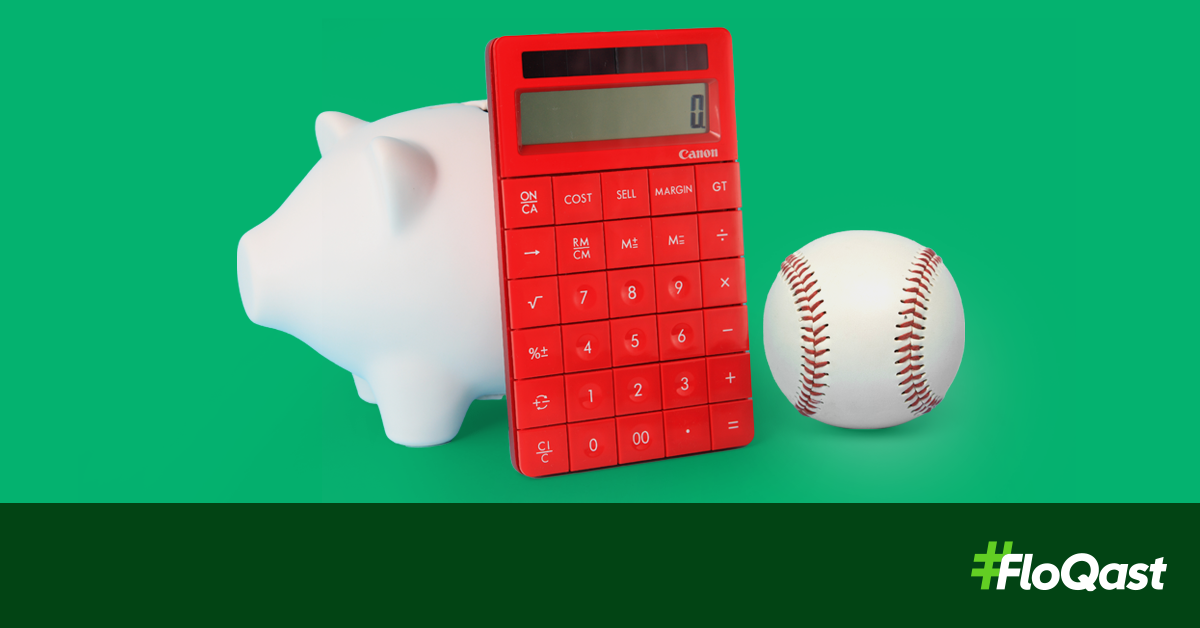
AI
AI at the Bat: Baseball as a Lens for Understanding AI’s Limitations and Potential
AI’s potential to revolutionize various industries, including accounting, is undeniable. Both its present capabilities and the general promise surrounding the technology can — and will — impact businesses broadly.
But far too often, we hear people painting in broad strokes, explaining things so generally that it’s clear they’re not totally sure what they’re talking about. Because AI is such a hot topic, and because of all that promise it possesses, I got together with my co-founders for a special episode of Blood, Sweat & Balance Sheets for an AI summit. In this episode, available for free, exclusively on FloQademy, we sought to discuss the intersection of AI and accounting, AI’s limitations and potential uses, and where there’s room for improvement.
To set the table and really understand where AI lives in a complicated landscape of LLMs, neural nets, machine learning, automation, and a plethora of other technologies, we decided to start with something familiar to us: Baseball.
I’m a fan. Mike is obsessed. But Cullen is not a sports person at all, so it took a group effort to find common ground. Check out the clip below for our discussion, and be sure to register for FloQademy for free to watch the whole episode and earn CPE/CPD.
The Basics of Machine Learning with a Baseball Twist
When it comes to machine learning, it’s all about data. Essentially, the more information you have, the better your predictions. In baseball, this means that the more games and runs you have data on, the more accurate your predictions about future performance will be. This is the essence of machine learning: using historical data to make predictions.
When discussing what constitutes “a lot” of data, Cullen emphasized that it’s relative to what you’re trying to predict.
“If you’re trying to predict a baseball player’s batting average, then you’re going to need every single one of his or her bats ever, and then maybe you can make good predictions,” he explained.
The more variables in your prediction, the more data you need. In machine learning, the more data you have, the better your prediction accuracy.
The Challenge of Aberrations in AI Predictions
One intriguing point we touched on was the impact of unforeseen changes on AI predictions. I gave an example of a baseball player who had laser eye surgery, drastically improving his batting average. Such aberrations challenge AI models, which rely on historical data to make predictions.
“If a model predicts a player will hit .240 based on past performance, but the player hits .300 post-surgery, the model’s predictions are thrown off. To me, that suggests a big problem for AI in the future. If there’s some variable outside of the data set that changes, does that then invalidate this massive data set that you’ve spent so much time building?”
If you’ve gotten this far, you deserve a treat that harkens back to the title. You’re welcome.
The Holy Grail: Generalization in AI
To answer that, Cullen went Biblical. Sorta. He explained that AI is really all about pattern matching. When an aberration occurs, it disrupts the pattern, causing AI models to falter. The ultimate goal in AI is generalization: creating models that can apply across various contexts and handle a wide range of situations.
“The holy grail of AI really is this idea of generalization,” he said. “If we can somehow, some way, shape, or form come up with a model that generalizes across a bunch of different topics and can make predictions about all kinds of things with a single model, or maybe multiple models sort of glued together, then you can account for a lot of different aberrations that might happen.”
This is why large language models (LLMs) like GPT are significant; they are generalized models that can handle diverse topics and tasks.
The Future of AI in Accounting
For AI to be truly effective, it needs access to all pertinent information. In Mike’s example, a generalized AI model should be able to read about the player’s laser eye surgery — so he can see the ball better — and adjust its predictions accordingly. This highlights the need for AI models to adapt and consider new data that may affect their predictions.
“The generalized model would need to be able to read an article about how this guy got laser eye surgery, contemplate that, and generate an appropriate response, like, ‘Hey, all this prior period data is somewhat invalid now. We need to accumulate more data before we can make any forecasts on this considering this development.’”
In the accounting world, this means customizing AI models to fit specific needs and continuously refining techniques to improve accuracy. Techniques like Retrieval-Augmented Generation (RAG), which preload AI with company-specific information, are promising. However, achieving nuanced answers remains complex.
Embracing AI’s Potential in Accounting
As AI continues to evolve, the accounting industry must balance leveraging its potential while considering its limitations. Customizing AI models to better fit specific accounting needs and refining techniques like RAG will be crucial steps forward.
As AI continues to evolve, the accounting industry must balance leveraging its potential while considering its limitations. Customizing AI models to better fit specific accounting needs and refining techniques like RAG will be crucial steps forward.
For a deeper dive into these topics and more, check out the full episode of Blood, Sweat & Balance Sheets on FloQademy. In this episode, we delve into the latest trends, challenges, and success stories of AI in the accounting world. Visit Learn.FloQast.com to register for free, watch the whole episode, and earn CPE/CPD credits.